LADICIM and IFCA rely on AI to automate the classification of graphite in iron foundries
Using convolutional neural networks, the new system identifies graphite morphology with high accuracy, overcoming the limitations of manual analysis and enabling more rigorous and efficient quality control
The correct classification of graphite in iron castings is essential to ensure that metal parts meet the high quality standards required by strategic sectors such as automotive, industrial machinery, energy and construction. Graphite, present in particulate form within the iron matrix, plays a crucial role in the mechanical and thermal properties of the material, which directly impacts its performance and durability in demanding applications.
For example, in the automotive industry, the use of nodular cast iron is crucial for the manufacture of components such as axles, engine blocks and suspension systems. The spherical shape of graphite in these applications improves the strength and ductility of the material, reducing stress concentration points and increasing the ability of parts to withstand mechanical loads and thermal shock. This extends vehicle life and reduces maintenance requirements, generating benefits for both manufacturers and end users.
Grey cast iron, on the other hand, is characterised by the presence of lamellar graphite, making it ideal for elements such as brake discs and cylinder heads, as it facilitates heat dissipation and vibration damping. This translates into greater energy efficiency and safer, quieter operation of equipment, improving industrial productivity and, consequently, benefiting the economy.
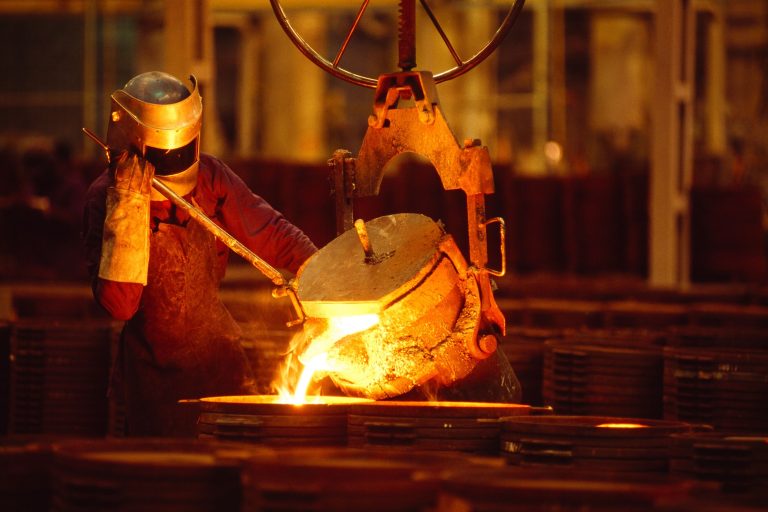
The proper classification of graphite shapes is also essential in construction, where castings are required to withstand varying loads and harsh environmental conditions. Vermicular graphite, with characteristics intermediate between nodular and lamellar, provides an ideal balance between strength and thermal conductivity, being optimal for components facing constant thermal and mechanical changes.
The ability to accurately identify graphite morphology in castings not only allows companies to fine-tune their production processes, but also ensures the manufacture of reliable and durable products. This has a positive impact on industrial sustainability by reducing resource consumption and waste generation, and contributes to the well-being of society by improving the safety and efficiency of the products we use every day. Thus, the correct classification of graphite is a pillar of innovation and competitiveness in various industries, affecting from the supply chain to the end user and promoting technological development that increases the quality of life.
Limitations of the traditional method of graphite classification
The current method of classifying graphite in iron castings is based on the visual observation of its microstructure by microscopy, in accordance with the UNE-EN ISO 945-1:2020 standard. This standard establishes six reference images, one for each of the families of cast irons, for an operator to classify, by visual comparison, the different forms of graphite particles present in cast iron samples. A procedure that depends on the experience and expertise of the analyst, who must identify morphological variations of graphite, such as lamellar, nodular or compact forms.
Despite its widespread use in industry, this method has notable limitations due to its qualitative nature. The classification is highly dependent on the subjective interpretation of the operator, which introduces a significant degree of variability in the results. Different analysts may reach divergent conclusions about the same microstructure, especially in cases of subtle transitions between graphite shapes.
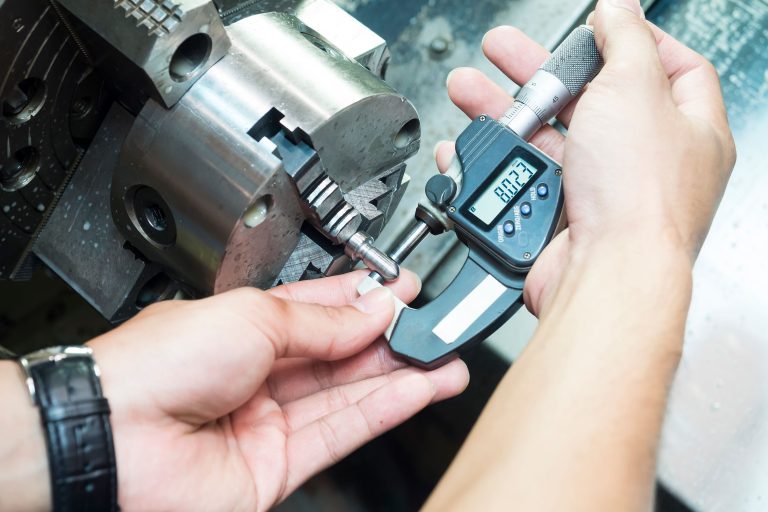
An added challenge for this system is the evaluation of samples with heterogeneous microstructures, where various forms of graphite coexist. In these cases, the operator must visually determine the relative proportion of each type of graphite, a complex and error-prone process. The need to evaluate each sample manually increases the time required for analysis, which in turn increases operating costs and reduces efficiency in high production demand contexts.
These limitations in the reliability and efficiency of the current system represent an obstacle to ensuring consistent material quality, a fact especially relevant in applications where the mechanical properties of castings must be accurate and stable. In this respect, the industry faces the challenge of adopting methods that maintain the accuracy of the analysis, while eliminating the biases and restrictions of the manual process.
Innovation in graphite sorting with Artificial Intelligence
In response to these challenges, the Laboratory of Materials Science and Engineering (LADICIM) of the University of Cantabria has developed, in collaboration with the Institute of Physics of Cantabria (IFCA-CSIC), an innovative study for the classification of graphite in iron castings, published in the journal Steel Research International. The research team has proposed a methodology that integrates artificial intelligence techniques, thus overcoming the limitations of traditional visual analysis.
The research, led by Marta Bárcena, Lara Lloret, Diego Ferreño and Isidro Carrascal, has enabled the development of a technique based on Convolutional Neural Networks (CNN) for the automatic classification of graphite microstructures from optical microscopy images. This approach relies on the use of deep neural networks, such as MobileNet and U-Net, to accurately and consistently analyse graphite morphology, overcoming the subjectivity inherent in manual analysis.
The study starts from a known reality: the classification of graphite shapes is essential for determining the mechanical properties of iron castings, but the traditional visual method has problems of repeatability and accuracy. LADICIM’s research has taken a significant step towards automating this process by using a deep learning approach, which analyses large volumes of visual data efficiently.
To make up for the lack of a sufficiently large dataset to allow adequate training of the algorithms, the research team created a synthetic dataset of images based on the reference micrographs provided by the UNE-EN ISO 945-1:2020 standard. This dataset includes 2,400 pure synthetic images (i.e. including only one type of graphite morphology among the six available families) and 1,500 mixed ones (each including two graphite morphologies), used to train and validate the CNN models.
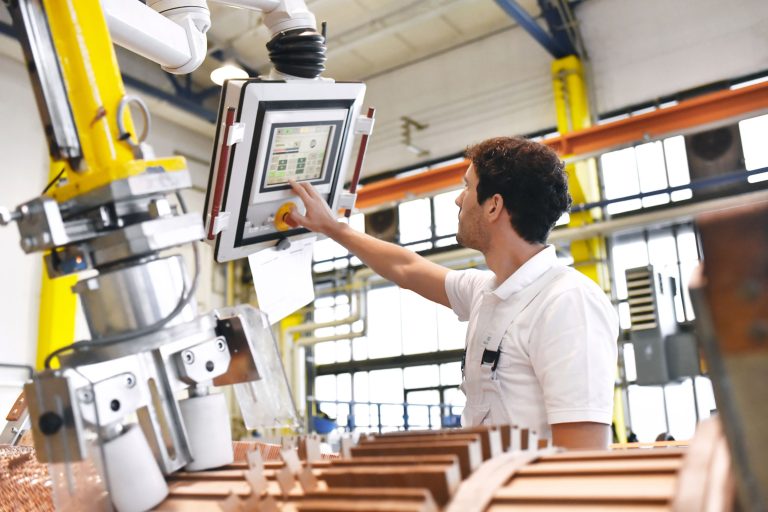
The MobileNet model focused on the classification of homogeneous images, while U-Net was used to segment the more complex mixed images by assigning labels to each pixel. This approach allows detailed identification of graphite shapes in complex samples, where multiple morphologies can coexist. The results achieved demonstrate that the algorithms are able to faithfully identify graphite features, enabling accurate classification with a hit rate of over 97% in pure images, and between 84% and 93% in mixed image segmentation.
In addition, the model showed a remarkable ability to process large amounts of data in a short time, a key feature for industrial applications demanding high efficiency.
An automated approach to real-time quality control
The development of this methodology represents a significant advance, enabling objective and repeatable analysis to ensure the quality of iron castings. The use of machine learning facilitates the scalability of the process and allows its integration into real-time quality control processes, improving traceability and optimising production.
The research developed by LADICIM and IFCA marks a turning point in the automated classification of graphite in castings. The collaboration between the two institutions has combined LADICIM’s expertise in materials science and microstructure characterisation with IFCA’s experience in advanced data processing and computational modelling. This synergy has been instrumental in creating a synthetic dataset that accurately emulates the characteristics of graphite according to ISO 945-1.
The integration of these techniques into quality control processes will improve the consistency of analysis, optimising the industrial production of castings. The proposed automated approach has the potential to be implemented in various industries, improving the efficiency and quality of production in sectors such as automotive, power generation and heavy machinery.